
Some researchers have used the GWR model and its variations to consider spatial varying characteristics that lie in the health risks factors of COVID-19. Global models are not so helpful for examining the effect of risk factors that may vary across space. However, one problem is that spatial epidemic data often exhibit high spatial heterogeneity. The studies above considered spatial autocorrelation from a global point of view. To solve the problem of spatial autocorrelation, many spatial models such as spatial error models (SEM) and spatial lag models (SLM) models have been utilized in the analysis of COVID-19. As the spread of infectious diseases is often spatially autocorrelated, traditional nonspatial statistical models are not so suitable because the data violates the independence criterion. However, in these studies, the spatial relationships among cities or countries were underestimated. Additionally, a number of researchers have estimated the association between environmental factors and COVID-19 transmission, specifically the influence of air quality, wind, humidity as well as temperature on COVID-19 cases.
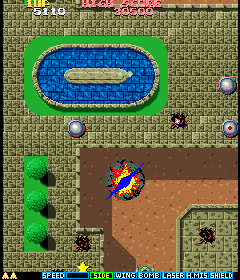
Factors such as population density, population mobility and accessibility to hospitals have also been considered in relation to the spread of COVID-19 in many studies. Some have found that COVID-19 has had a more significant impact on poor areas while a few have found that the influence of income has not been significant in certain study areas.
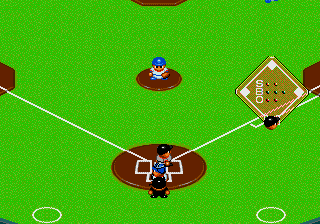
Researchers have explored the relationship between socioeconomic as well as infrastructural factors and the spread of the COVID-19 virus. It is hoped that these findings improve public and governmental awareness of potential health risks and therefore influence epidemic control strategies. The yielding ESF-SVC coefficients could also provide an intuitive method for discovering the different impacts of influencing factors across space in large study areas. This study could provide a feasible path to improve the model fit by considering the problem of spatial autocorrelation and heterogeneity that exists in COVID-19 modeling. The temperature showed mixed correlation as time passed, with positive and negative coefficients at 2.42 ☌ and 8.17 ☌, respectively. The influence of migration score was less contributive and less significant in cities around Wuhan than cities further away, while altitude showed a stronger contribution to the decrease of infection rates in high altitude cities. The impact of health risk factors varied as the study extent changed: In Hubei province, only population density and wind speed showed significant spatially constant impact while in mainland China, other factors including migration score, building density, temperature and altitude showed significant spatially varying impact. The ESF-SVC was able to take good control of over-fitting problems compared with ordinary least square (OLS), eigenvector spatial filtering (ESF) and geographically weighted regression (GWR) models, with a higher adjusted R 2 and lower cross validation RMSE. An eigenvector spatial filtering-based spatially varying coefficient model (ESF-SVC) was developed to find out how the influence of selected health risk factors varies across space and time.
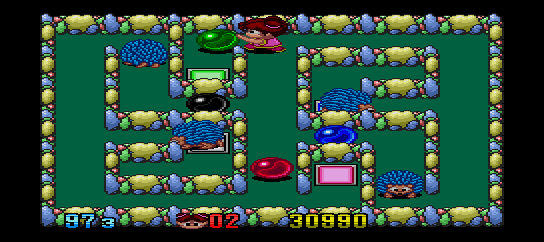
The purpose of this paper was to explore how selected health risk factors are related to the pandemic infection rate within different study extents and to reveal the spatial varying characteristics of certain health risk factors. Several studies have examined the effect of corresponding health risk factors in different places, but the problem of spatial heterogeneity has not been adequately addressed. The COVID-19 pandemic has led to many deaths and economic disruptions across the world.
